It’s scary enough making a doctor’s appointment to see if a strange mole could be cancerous. Imagine, then, that you were in that situation while also living far away from the nearest doctor, unable to take time off work and unsure you had the money to cover the cost of the visit. In a scenario like this, an option to receive a diagnosis through your smartphone could be lifesaving.
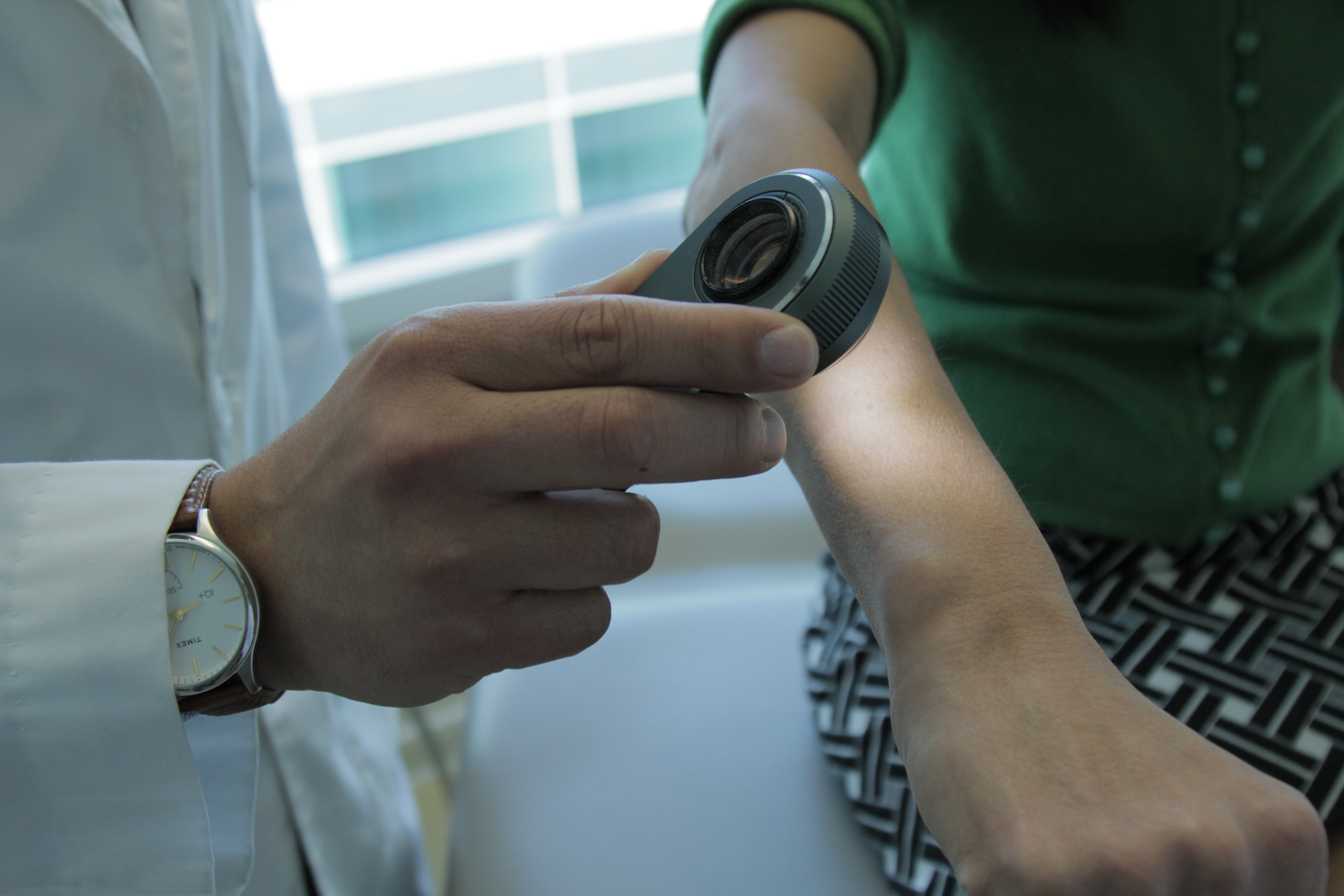
A dermatologist using a dermatoscope, a type of handheld microscope, to look at skin. Computer scientists at Stanford have created an artificially intelligent diagnosis algorithm for skin cancer that matched the performance of board-certified dermatologists. (Image credit: Matt Young)
«We realized it was feasible, not just to do something well, but as well as a human dermatologist," said Sebastian Thrun, an adjunct professor in the Stanford Artificial Intelligence Laboratory. «That’s when our thinking changed. That’s when we said, ‘Look, this is not just a class project for students, this is an opportunity to do something great for humanity.’»
The final product, the subject of a paper in the Jan. 25 issue of Nature, was tested against 21
Why skin cancer
Every year there are about 5.4 million new cases of skin cancer in the United States, and while the
Diagnosing skin cancer begins with a visual examination. A dermatologist usually looks at the suspicious lesion with the naked eye and with the aid of a dermatoscope, which is a handheld microscope that provides
Bringing this algorithm into the examination process follows a trend in computing that combines visual processing with deep learning, a type of artificial intelligence modeled after neural networks in the brain. Deep learning has a
«We made a very powerful machine learning algorithm that learns from data," said Andre Esteva,
The algorithm was fed each image as raw pixels with an associated disease label. Compared to other methods for training algorithms, this one requires very little processing or sorting of the images prior to classification, allowing the algorithm to work off a wider variety of data.
From cats and dogs to melanomas and carcinomas
Rather than building an algorithm from scratch, the researchers began with an algorithm developed by Google that was already trained to identify 1.28 million images from 1,000 object categories. While it was primed to be able to differentiate cats from dogs, the researchers needed it to know a malignant carcinoma from a benign seborrheic keratosis.
«There’s no huge dataset of skin cancer that we can just train our algorithms on, so we had to make our own," said Brett Kuprel,
After going through the necessary translations, the researchers collaborated with dermatologists at Stanford Medicine, as well as Helen M. Blau, professor of microbiology and immunology at Stanford and
During testing, the researchers used only
The algorithm’s performance was measured through the creation of a
An added advantage of the algorithm is that, unlike a person, the algorithm can be made more or less sensitive, allowing the researchers to tune its response depending on what they want it to assess. This ability to alter the sensitivity hints at the depth and complexity of this algorithm. The underlying architecture of seemingly irrelevant photos — including cats and dogs — helps it better evaluate the skin lesion images.
Health care by smartphone
Although this algorithm currently exists on a computer, the team would like to make it smartphone compatible in the near future, bringing reliable skin cancer diagnoses to our fingertips.
«My main eureka moment was when I realized just how ubiquitous smartphones will be," said Esteva. «Everyone will have a supercomputer in their pockets with a number of sensors in it, including a camera. What if we could use it to visually screen for skin cancer? Or other ailments?»
The team believes it will be relatively easy to transition the algorithm to mobile devices but there still needs to be further testing in a
«Advances in
Even in light of the challenges ahead, the researchers are hopeful that deep learning could someday contribute to visual diagnosis in many medical fields.
Additional Stanford